The Biggest AI Trends to Watch in 2025: What You Need to Know
Last Updated: May 4, 2025
Artificial Intelligence isn't just evolving; it's accelerating at a breakneck pace. What seemed like science fiction only a few years ago is rapidly becoming integrated into our daily lives and work. Staying informed about the key AI trends isn't just for tech enthusiasts anymore – it's crucial for professionals, businesses, and anyone looking to navigate the future effectively. The advancements we're seeing are reshaping industries, creating new opportunities, and demanding new skills.
From AI systems that can act autonomously to models that understand images and sound as well as text, the landscape is shifting dramatically. Understanding these trends helps you anticipate changes, leverage new tools, and make informed decisions. This guide explores the 10 most significant AI trends poised to make a major impact in 2025, providing insights into what they mean and how you can prepare.
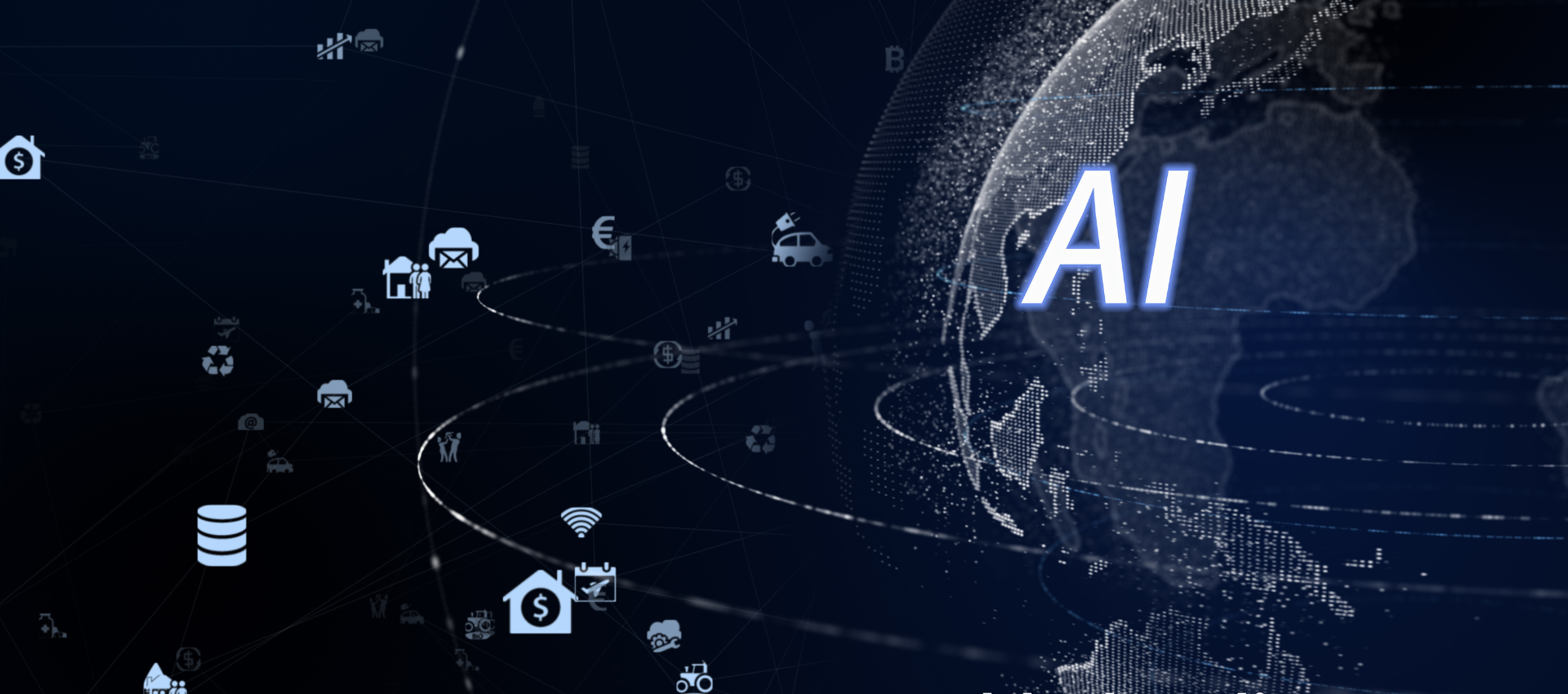
Why Understanding AI Trends Matters
Keeping up with AI trends is more than just satisfying curiosity. It has tangible implications:
- Career Impact: Understanding AI trends helps identify emerging job roles and skills in demand, allowing you to adapt your career path proactively. It also highlights how AI might augment or change your current role.
- Business Strategy: Businesses need to understand AI trends to identify opportunities for innovation, efficiency gains, competitive advantages, and potential disruptions in their market.
- Informed Decision-Making: From choosing software tools to understanding societal changes, awareness of AI trends empowers better personal and professional decisions.
- Ethical Awareness: Many trends raise important ethical questions. Staying informed allows for participation in crucial discussions about responsible AI development and deployment.
- Leveraging Opportunities: Recognizing trends early allows individuals and organizations to adopt new AI tools and techniques effectively, gaining a first-mover advantage.
In short, AI is becoming foundational technology. Understanding its trajectory is essential for navigating the modern world.
Key AI Trends Shaping 2025
1. The Rise of Agentic AI Systems
Beyond simply responding to prompts, AI is becoming more autonomous. Agentic AI systems, or AI agents, are designed to achieve complex goals by breaking them down into smaller steps, using tools (like web browsers or code interpreters), interacting with software, and even collaborating with other agents. Think of them as digital assistants capable of executing multi-step tasks with minimal human intervention.
Implications:
- Significant potential for automating complex workflows in business, research, and personal productivity.
- New possibilities for personalized services and proactive assistance.
- Increased need for robust safety protocols and human oversight mechanisms.
- Potential disruption to tasks currently requiring significant human coordination.
Internal Link: These agents are poised to supercharge AI automation tools, enabling more sophisticated task execution.
[Visual Placeholder: Diagram showing an AI agent receiving a goal, breaking it into steps, using tools (browser, calculator), and producing a result]
2. Multimodal AI Becomes Mainstream
AI is breaking free from text-only interactions. Multimodal models can understand, process, and generate information across different types of data, including text, images, audio, and even video. Models like Google's Gemini and OpenAI's GPT-4o exemplify this trend, allowing users to interact using voice, upload images for analysis, or generate images from text descriptions.
Implications:
- More natural and intuitive human-AI interaction (e.g., voice commands, visual queries).
- Enhanced capabilities in areas like content creation, data analysis, accessibility tools, and robotics.
- New applications combining different data types (e.g., generating descriptions for images, summarizing videos).
- Increased complexity in model training and evaluation.
Internal Link: Explore how to leverage these capabilities in our guides for Gemini AI and ChatGPT.
[Visual Placeholder: Split graphic showing text, image, and audio icons pointing towards an AI model, which then outputs text, images, or audio]
3. Small Language Models (SLMs) Gain Traction
While massive Large Language Models (LLMs) like GPT-4 dominate headlines, there's a growing trend towards smaller, more efficient models. SLMs are designed to perform specific tasks effectively while requiring significantly less computational power and data. This makes them suitable for deployment on edge devices (like smartphones), specialized applications, and scenarios where cost or latency is a major concern.
Implications:
- Wider deployment of AI capabilities on personal devices and in resource-constrained environments.
- Lower costs for developing and running certain AI applications.
- Potential for faster response times in specific tasks.
- Increased focus on optimizing models for efficiency alongside capability.
[Visual Placeholder: Simple graphic comparing a large LLM icon (large size, high cost) vs. a small SLM icon (small size, low cost)]
4. Focus on AI Reasoning and Reliability
As AI takes on more critical tasks, ensuring its reliability and improving its reasoning capabilities are paramount. Current models can sometimes produce plausible-sounding but incorrect information (known as "hallucinations"). Research and development are increasingly focused on enhancing AI's ability to perform logical reasoning, verify facts, explain its conclusions, and operate more reliably, especially in high-stakes domains like healthcare or finance.
Implications:
- Increased trustworthiness and adoption of AI in critical applications.
- Development of techniques for fact-checking and grounding AI outputs in reliable sources.
- Better explainability, allowing users to understand *how* an AI reached a conclusion.
- Continued challenges in completely eliminating inaccuracies.
[Visual Placeholder: Abstract graphic representing gears turning or a logic circuit, symbolizing reasoning]
5. Custom Silicon and Hardware Acceleration
Training and running large AI models requires immense computational power. This has spurred a trend towards developing specialized hardware (like Google's TPUs, NVIDIA's GPUs optimized for AI, and custom chips from various companies) designed specifically for AI workloads. This hardware acceleration is crucial for making advanced AI feasible and more efficient.
Implications:
- Faster training times for new AI models.
- More efficient and lower-cost inference (running trained models).
- Enabling more complex AI models to be developed and deployed.
- Intensified competition and innovation in the semiconductor industry.
[Visual Placeholder: Image of a modern AI accelerator chip or GPU]
6. AI Integration in Scientific Discovery
AI is becoming an indispensable tool in scientific research across various fields. From accelerating drug discovery by predicting protein structures (like DeepMind's AlphaFold) to analyzing vast datasets in climate modeling, particle physics, and astronomy, AI is helping scientists tackle complex problems, identify patterns, and generate hypotheses at an unprecedented scale.
Implications:
- Faster pace of scientific breakthroughs and innovation.
- Ability to analyze complex datasets that were previously intractable.
- New research methodologies combining AI simulation and experimental data.
- Increased demand for scientists with AI and data science skills.
[Visual Placeholder: Abstract graphic representing data analysis, DNA helix, or particle collisions]
7. Intensified Focus on AI Ethics, Governance, and Regulation
As AI becomes more powerful and pervasive, concerns about its ethical implications, potential misuse, and societal impact are growing. This is leading to an intensified focus on developing ethical guidelines, establishing governance frameworks, and implementing regulations. Key areas include bias mitigation, transparency, accountability, safety, and data privacy.
Implications:
- Development of industry standards and best practices for responsible AI.
- Introduction of new regulations and compliance requirements for AI systems (e.g., EU AI Act).
- Increased public and governmental scrutiny of AI deployment.
- Growing importance of roles focused on AI ethics and governance within organizations.
[Visual Placeholder: Graphic representing balance scales labeled 'Innovation' and 'Ethics' or a shield icon]
8. Hyper-Personalization Driven by AI
AI's ability to analyze vast amounts of user data is enabling unprecedented levels of personalization in various domains. From hyper-targeted advertising and personalized product recommendations on e-commerce sites to customized learning paths in education and tailored content feeds on social media, AI is creating experiences uniquely adapted to individual preferences and behaviors.
Implications:
- More relevant and engaging user experiences across digital platforms.
- Increased effectiveness of marketing and sales efforts.
- Potential concerns regarding data privacy, filter bubbles, and manipulation.
- Demand for AI systems that can understand user context and preferences deeply.
[Visual Placeholder: Graphic showing different personalized content streams flowing towards individual user icons]
9. Enterprise Adoption and ROI Focus
While initial AI adoption in businesses often involved experimentation, the focus is shifting towards demonstrating tangible return on investment (ROI). Companies are moving beyond pilot projects to integrate AI more deeply into core business processes, seeking measurable improvements in efficiency, cost reduction, revenue generation, or customer satisfaction. This requires a more strategic approach to AI implementation.
Implications:
- Increased demand for AI solutions with clear business value propositions.
- Greater emphasis on measuring the impact and ROI of AI initiatives.
- Need for skills in integrating AI into existing enterprise systems and workflows.
- Growth of AI platforms and tools specifically designed for enterprise use cases.
[Visual Placeholder: Simple bar chart showing increasing enterprise AI adoption or ROI over time]
10. Addressing AI's Environmental Footprint
The significant energy consumption required for training and running large AI models has raised concerns about their environmental impact. Consequently, there's a growing trend focused on developing more energy-efficient AI algorithms, optimizing hardware usage, and exploring sustainable practices for AI development and deployment (often referred to as Green AI).
Implications:
- Increased research into energy-efficient model architectures and training techniques.
- Development of metrics and standards for measuring the carbon footprint of AI systems.
- Greater consideration of environmental impact in choosing AI solutions.
- Potential trade-offs between model performance and energy efficiency.
[Visual Placeholder: Graphic combining an AI brain/chip icon with a green leaf or recycling symbol]
How to Prepare for These AI Trends
Navigating these trends requires proactive adaptation:
For Individuals:
- Embrace Lifelong Learning: Stay curious and continuously learn about AI developments through reputable sources, courses, and hands-on experimentation.
- Develop AI Literacy: Understand basic AI concepts, capabilities, and limitations, even if you're not in a technical role.
- Focus on Human-Centric Skills: Cultivate skills that AI struggles with, such as critical thinking, creativity, emotional intelligence, complex problem-solving, and collaboration.
- Learn to Work *with* AI: Practice using AI tools relevant to your field. Mastering prompt engineering and learning how to leverage AI as an assistant are becoming crucial skills.
For Businesses:
- Develop an AI Strategy: Identify specific business problems where AI can provide value and align AI initiatives with overall business goals.
- Prioritize Ethical Considerations: Establish clear guidelines for responsible AI use, addressing bias, transparency, and privacy from the outset.
- Invest in Workforce Training: Upskill and reskill employees to work effectively alongside AI tools and understand their implications.
- Start Small and Iterate: Begin with pilot projects to test AI solutions and demonstrate value before scaling up complex implementations.
- Stay Agile: The AI landscape changes quickly; be prepared to adapt strategies and adopt new tools as the technology evolves.
Frequently Asked Questions (FAQ)
It's difficult to single out one, as many trends are interconnected. However, the rise of Agentic AI and the mainstream adoption of Multimodal AI are likely to have very broad and noticeable impacts on how we interact with technology and automate tasks in the near term.
The pace is incredibly fast, often exceeding predictions. Major advancements in model capabilities, hardware, and applications are frequently announced. What seems cutting-edge today might become standard within 6-12 months. This rapid pace underscores the importance of continuous learning.
Risks are multifaceted and include potential job displacement due to automation, the amplification of bias present in training data, the spread of misinformation generated by AI, data privacy violations, security vulnerabilities, and the ethical challenges of increasingly autonomous systems. Addressing these requires careful governance, regulation, and responsible development practices.
The opportunities are vast, including significant boosts in productivity and efficiency across industries, acceleration of scientific discovery, creation of new forms of personalized services and entertainment, improved accessibility for people with disabilities, and the potential to help solve complex global challenges like climate change and disease.
Absolutely not. While some trends involve coding (like AI coding assistants), many powerful AI tools are designed for non-technical users. AI writing assistants, meeting summarizers, presentation builders, and workflow automation platforms often require little to no coding knowledge. The key skill is often learning how to communicate your needs effectively to the AI (prompting).
Follow reputable AI research labs (like OpenAI, Google DeepMind, Meta AI), major tech news outlets, specialized AI newsletters, academic publications (like arXiv for preprints), and industry conferences. Experimenting directly with new AI tools and platforms as they become available is also a great way to stay current. And of course, keep checking back here at AI Tech Insights!